Medical Knowledge Synthesis
Medical evidence is currently disseminated mostly through unstructured or semi-structured free-text documents, e.g., clinical guidelines, systematic reviews or primary research articles. Turning these text documents into structured, linked data through NLP would allow users to reason and answer questions about the body of evidence as a whole, such as:
- Is there a disagreement between latest research articles and clinical guideline recommendations?
- How long does translation of clinical research into practice actually take?
- Do multiple publications report the same data and should be considered duplicates in a systematic review?
- Is a trial report consistent with the initial trial synopsis?
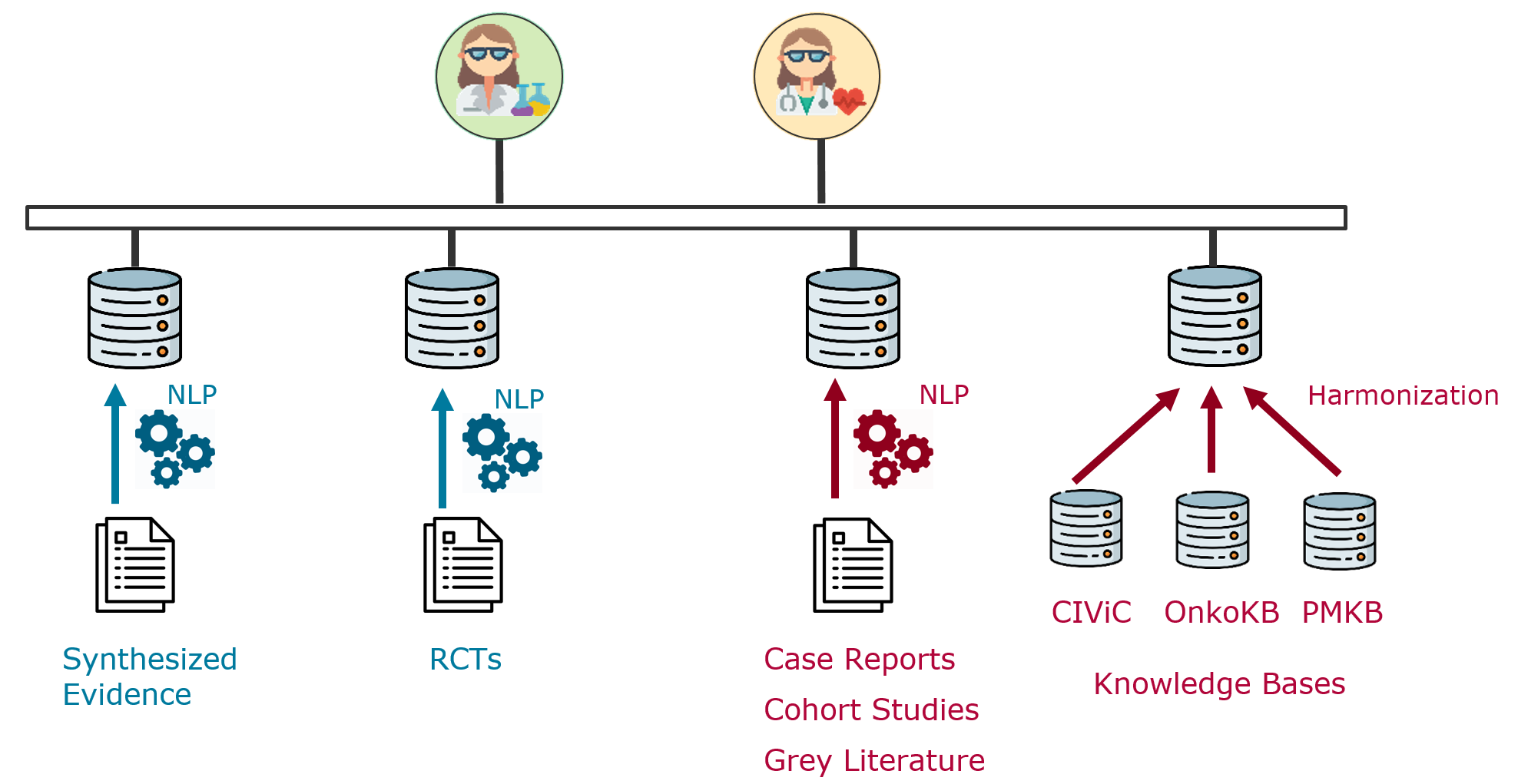
Links
Technologies
References
Florian Borchert, Laura Meister, Thomas Langer, Markus Follmann, Bert Arnrich, and Matthieu-P. Schapranow. Controversial Trials First: Identifying Disagreement Between Clinical Guidelines and New Evidence, Proceedings of the AMIA Annual Symposium, pp. 237-246, San Diego, USA (2021) 🏆 Distinguished Paper Award [Link]
Florian Borchert*, Andreas Mock*, Aurelie Tomczak*, Jonas Hügel, Samer Alkarkoukly, Alexander Knurr, Anna-Lena Volckmar, Albrecht Stenzinger, Peter Schirmacher, Jürgen Debus, Dirk Jäger, Thomas Longerich, Stefan Fröhling, Roland Eils, Nina Bougatf, Ulrich Sax, Matthieu-P Schapranow. Knowledge Bases and Software Support for Variant Interpretation in Precision Oncology, Briefings in Bioinformatics, Volume 22, Issue 6, November 2021, bbab134 (* equal contribution) IF = 11.6